A specific, meticulously organized approach to problem-solving, often employed in data analysis, may involve a phased process. This framework might include well-defined steps, checkpoints, and clear criteria for progressing through various stages of a project. For instance, an iterative process might consist of data collection, data cleaning, model selection, and validation, each with its own set of metrics and success criteria.
Such structured problem-solving methodologies are invaluable in ensuring a consistent, high-quality outcome, minimizing error rates, and enabling reproducibility of results. The benefits include increased efficiency and accountability throughout the project lifecycle. A well-defined process, coupled with clear communication and documentation, facilitates better collaboration among team members. The reliability and predictability they bring contribute significantly to robust data analysis. In essence, the methodology serves as a blueprint for successful data analysis projects, guaranteeing a higher likelihood of producing impactful insights.
This discussion of structured problem-solving forms the cornerstone for a deeper dive into the methodologies of data analysis. The following sections will explore specific techniques and their practical applications in different domains.
Dane Dash
A structured approach to problem-solving in data analysis is crucial for reliable results. This framework ensures consistency and minimizes errors.
- Iterative process
- Data collection
- Validation metrics
- Clear criteria
- Reproducibility
- Team collaboration
- Data quality
The key aspects outlined above demonstrate the comprehensive nature of a structured approach. Iterative processes, for example, involve repeated cycles of data collection, analysis, and validation. Clear criteria ensure consistency in these cycles. High-quality data is essential, impacting the validity of analysis. Reproducibility, driven by clear methodologies and documentation, increases reliability. The importance of team collaboration underpins the execution of a structured process. A well-defined, documented, and consistently applied methodology enhances the efficiency and quality of data analysis, contributing to reliable outcomes.
1. Iterative Process
An iterative process, a fundamental aspect of structured problem-solving, is intrinsically linked to a data-driven approach, often incorporating elements crucial to a comprehensive methodology. This iterative nature is reflected in the cyclical nature of data analysis, where each cycle builds upon the previous one, refining the understanding of the problem and the resulting solution.
- Defining the Scope and Objectives:
Clearly defining the project's scope and objectives establishes the initial parameters. This step ensures alignment between the problem definition and the subsequent analysis. A well-defined scope is crucial in focusing resources and establishing measurable criteria for evaluating results. For example, a marketing campaign might initially focus on website traffic, later expanding to consider conversion rates and customer demographics as those aspects become more significant.
- Data Gathering and Analysis:
Gathering relevant data, crucial for supporting the scope and objectives, often involves multiple iterations to ensure data quality and completeness. This phase might include initial data collection, followed by cleaning and validation, and subsequent adjustments based on emerging insights. For instance, initial customer surveys may reveal gaps in data coverage, prompting further data collection and adjustment of the analysis approach.
- Model Refinement and Validation:
Developing and refining analytical models is an iterative process. Initial models might yield preliminary results that trigger further investigation into assumptions or data limitations. Validation steps and feedback loops are key elements that guide the refinements. For example, a predictive model for customer churn might be revised multiple times based on feedback from the initial model's results, leading to improved accuracy.
- Communication and Feedback Loops:
Effective communication and feedback mechanisms are vital during each iteration. Progress reports and presentations allow stakeholders to understand the analysis's trajectory, enabling adjustments or redirection. Regular communication ensures that the solution aligns with evolving needs and goals. Feedback from stakeholders, for instance, might suggest a need to re-focus efforts on a specific aspect of the analysis.
These iterative steps, collectively, contribute to the robustness and reliability of the overall data analysis. Each cycle of data gathering, analysis, model refinement, and feedback allows for continuous improvement and adaptation to new insights, resulting in a more comprehensive and accurate understanding of the issue at hand. This iterative process, central to a well-structured methodology, directly supports the overarching aims of data-driven problem-solving.
2. Data Collection
Data collection is a fundamental component of any structured problem-solving approach, including those employing a methodical framework for data analysis. Its quality and appropriateness directly impact the validity and reliability of subsequent analysis, making it a critical initial step within a data-driven methodology. A robust data collection strategy is integral to achieving reliable results.
- Defining Data Needs:
Clear identification of the specific data required for the analysis is paramount. This necessitates careful consideration of the problem's nature and objectives. Determining the scope and necessary parameters for gathering relevant data is essential. For example, understanding the target population, variables to be measured, and desired level of detail in the dataset are vital steps. Failure to accurately define requirements can lead to data gaps or redundancies later.
- Choosing Data Sources:
Selection of appropriate data sources is crucial. The chosen sources must be reliable, accessible, and representative of the target population. Consideration must be given to data accuracy, potential biases, and limitations of each source. For instance, using outdated or incomplete databases can compromise the integrity of findings. Selecting diverse and reputable sources, like government surveys, industry reports, and user-generated content, offers a more complete and balanced perspective, leading to more accurate conclusions.
- Data Collection Methods:
Selection of appropriate methodologies for collecting data is crucial. Methods should be aligned with data needs and maintain quality standards. Consider whether to employ surveys, experiments, observations, or existing databases to ensure the data gathered aligns with project aims and objectives. Maintaining consistent application of the chosen method across the entire data collection process ensures data quality. For instance, using consistent survey questions and procedures across the target population guarantees consistent data points.
- Data Validation and Cleaning:
Ensuring data quality and integrity throughout the process is crucial. Implementing validation procedures and data cleaning steps are essential. This involves checking for inconsistencies, missing values, and outliers to maintain data accuracy and minimize potential errors in subsequent analysis. Identifying and handling errors at this stage can drastically improve the reliability of the insights derived from the data.
Effective data collection forms the foundation of any analysis. By methodically defining needs, selecting relevant sources, employing appropriate collection techniques, and meticulously validating the gathered data, the reliability and validity of subsequent analyses are significantly enhanced. This, in turn, strengthens the credibility of any conclusions drawn from the data. A well-structured data collection strategy, implemented as part of a more comprehensive problem-solving framework, directly supports the overall objectives of achieving reliable and impactful findings.
3. Validation Metrics
Validation metrics are indispensable components of a structured problem-solving approach, playing a pivotal role in ensuring the reliability and accuracy of analysis. Within a robust data-driven framework, they serve as benchmarks, enabling assessment of the effectiveness and validity of the chosen approach. Rigorous validation, utilizing appropriate metrics, directly influences the quality of results, and their applicability in real-world contexts. A well-defined set of validation metrics supports the iterative nature of the process, ensuring that analysis consistently converges toward the desired solution.
The importance of validation metrics extends to many fields. In medical research, validating the efficacy of a new drug involves meticulous metrics assessing patient outcomes, side effects, and response rates. Similarly, in financial modeling, validation metrics are used to assess the accuracy of models predicting market trends. Correctly applied metrics quantify the model's fit to historical data and its predictive power in future scenarios. Such rigorous validation ensures the models are reliable tools for decision-making. Furthermore, in social science research, metrics like survey response rates and sample representativeness are essential to verify the validity of findings.
Effective validation metrics enable the identification of potential errors or biases early in the process. By comparing model outputs against established standards or benchmarks, practitioners can pinpoint areas requiring further investigation or adjustment. This allows the refinement of methods and procedures, ultimately leading to more robust conclusions. The ongoing evaluation through validation metrics reinforces the structured nature of the approach, ensuring each stage aligns with the overall objectives. Moreover, well-defined metrics contribute to transparency and reproducibility in the analysis process, facilitating wider review and validation by others, thus strengthening the trustworthiness and reliability of the results. Ultimately, the application of appropriate validation metrics underpins the credibility of any data-driven initiative, ensuring its efficacy and utility in real-world applications.
4. Clear Criteria
Clear criteria are fundamental to a structured problem-solving approach, particularly in data analysis. They define the boundaries and expectations for each stage of the process. Without clearly defined criteria, progress becomes ambiguous, potentially leading to inconsistent results, wasted effort, and inaccurate conclusions. A lack of clear criteria undermines the iterative process, impeding the ability to refine and improve solutions effectively.
Consider a marketing campaign aimed at increasing website traffic. Clear criteria are essential. Defining "success" solely by website visits lacks depth. Instead, metrics like unique visitors, bounce rate, time on site, and conversion rates provide a more nuanced understanding of the campaign's effectiveness. Without these specific, measurable criteria, evaluating the campaign's success or adjusting strategy becomes problematic, as progress becomes less easily trackable. The same applies to any complex data analysis project, where explicit benchmarks are crucial to assess each stage and iterate towards the optimal outcome.
The practical significance of clear criteria within a structured problem-solving approach is considerable. They ensure consistency and reproducibility, enabling others to replicate the analysis and validate findings. The ability to define specific metrics also allows for a more objective evaluation of progress, leading to better decision-making. Moreover, this clarity minimizes the risk of bias or subjectivity creeping into the analysis process, ultimately resulting in more reliable and trustworthy results. These clear criteria are not merely a component of the methodology; they are critical to the structure and integrity of the entire process, driving the analysis toward a meaningful and impactful solution.
5. Reproducibility
Reproducibility, a cornerstone of rigorous data analysis, is intrinsically linked to the efficacy of any structured problem-solving methodology. A process designed for reproducibility inherently facilitates the validation and replication of findings. A structured approach, often employed in data-driven problem-solving frameworks, inherently necessitates the meticulous documentation of methods, procedures, and data, creating conditions for reproducibility. Clear guidelines, standardized protocols, and detailed documentation of steps taken are crucial components of a reproducible process.
Consider a scientific study aimed at validating a new drug. Reproducibility is paramount. Detailed protocols, including experimental design, sample preparation, measurement techniques, and data analysis methods, must be documented meticulously. This allows other researchers to precisely replicate the study and verify the results. Without this emphasis on reproducibility, the reliability of findings is compromised. Similarly, in business, consistent application of an analytical model across multiple projects ensures consistency and accurate predictions, demonstrating the practical application of reproducibility to produce reliable and comparable results. If the approach is not meticulously documented and repeatable, the value of the analysis is significantly diminished.
The practical significance of understanding this connection is substantial. Reproducible analysis fosters trust in results, enabling wider verification and validation. Robust documentation safeguards against errors and biases, enhancing the reliability of conclusions drawn. It facilitates collaboration among researchers and practitioners by providing a common ground for evaluation and advancement of knowledge. The importance of reproducibility in data analysis extends beyond scientific research, impacting diverse fields like business analytics, financial modeling, and public policy. By emphasizing reproducibility, a commitment to reliable and trustworthy results, aligned with a structured approach to problem-solving, is demonstrably strengthened. Ultimately, the capability of reliably replicating a process underpins the credibility of any analysis and reinforces its relevance in real-world applications. Challenges remain in ensuring reproducibility across diverse teams and contexts. However, the fundamental link between reproducibility and a reliable data-driven framework remains crucial for producing meaningful results.
6. Team Collaboration
Effective team collaboration is essential for any structured problem-solving approach, particularly within the context of data-driven methodologies. A cohesive team, working in concert, enhances the quality and efficiency of analysis, amplifying the value of a structured approach to problem-solving. This collaboration translates into a more robust framework for data-driven decisions, producing reliable and actionable insights.
- Shared Understanding of Objectives:
A shared understanding of project goals and criteria is fundamental. Clear communication of objectives, along with established success metrics, ensures that all team members are aligned. This shared understanding promotes consistent effort and avoids misinterpretations, ultimately leading to a unified approach that strengthens the data analysis process. Divergent interpretations can lead to errors and inconsistencies, which undermines the credibility and efficacy of the analytical process. Misaligned goals may result in wasted resources.
- Division of Labor and Expertise:
Effective division of tasks based on individual expertise maximizes efficiency. Assigning roles according to skill sets ensures that specialized knowledge is applied effectively. This allows tasks to be handled efficiently, reduces redundancy, and fosters knowledge sharing. Specialization of tasks ensures that critical elements are not overlooked. Effective knowledge sharing fosters a dynamic and adaptive response to challenges and promotes innovation.
- Open Communication and Feedback Loops:
Facilitating open communication channels and establishing clear feedback loops is critical. This promotes a collaborative environment, where team members can share insights and concerns. Prompt feedback mechanisms allow for the rapid identification of potential roadblocks or errors in the process, enabling timely adjustments. Transparent communication prevents misinterpretations and fosters a sense of shared ownership over the project. Suppressed communication may lead to errors or the overlooking of potential solutions.
- Conflict Resolution and Dispute Management:
Team collaboration requires mechanisms for managing conflicts constructively. A structured approach to conflict resolution, fostering respect for differing perspectives, ensures a harmonious working environment. This structured process allows for the fair consideration of various viewpoints, preventing stagnation, and facilitating productive dialogue that leads to better outcomes. A lack of these mechanisms may escalate minor conflicts into major impediments to progress.
In summary, team collaboration is integral to the success of a structured data analysis process. These facets, when effectively integrated, ensure that a team effectively navigates the intricacies of problem-solving, fostering consistency, maximizing the utilization of specialized expertise, and ensuring the integrity of findings. This approach promotes not only efficiency but also fosters a culture of shared knowledge and collective success, which is essential for a structured problem-solving methodology.
7. Data Quality
Data quality is a foundational element within any structured problem-solving approach, including those employing a methodical framework. The quality of data directly impacts the reliability and validity of subsequent analysis. Poor data quality can lead to inaccurate conclusions, flawed models, and ultimately, ineffective solutions. A strong emphasis on data quality is not just a best practice, it's a necessity in a reliable framework for analysis.
Data quality issues can manifest in various ways, including inaccuracies, inconsistencies, incompleteness, and irrelevancy. These imperfections can undermine the entire analysis process. For example, in a market research study, incomplete customer data might skew insights regarding purchasing habits. In a medical trial, inaccurate data entries could invalidate the results and lead to inaccurate conclusions about treatment efficacy. These examples demonstrate the direct correlation between data quality and the integrity of subsequent analysis. A flawed dataset will inevitably yield flawed interpretations, highlighting the significance of meticulously assessing and refining data quality.
The practical significance of understanding this connection is substantial. A structured approach to problem-solving cannot overlook the inherent link between data quality and solution effectiveness. Consequently, robust procedures for data validation, cleaning, and verification are integral to a reliable framework. Addressing issues like data entry errors, inconsistencies in measurement, or missing values early in the process will ultimately improve the reliability and validity of any resulting analyses and models. Prioritizing data quality directly reinforces the efficacy of any data-driven initiative by ensuring the input is sound and thus, the output can be trusted. A rigorous data quality assurance process strengthens the foundation of any structured problem-solving approach, creating a pathway to dependable and insightful conclusions.
Frequently Asked Questions (FAQ) - Structured Problem-Solving
This FAQ section addresses common inquiries regarding structured problem-solving methodologies, particularly in the context of data-driven analysis. Understanding these questions and answers can clarify the application and benefits of these frameworks.
Question 1: What distinguishes a structured approach to problem-solving from ad-hoc methods?
A structured approach employs a predefined, repeatable process. This contrasts with ad-hoc methods, which lack a formal framework. Structured methodologies offer increased consistency, reducing errors and improving the reliability of results. Clear steps and criteria ensure a more efficient and methodical resolution to problems. The structured approach allows for documentation, repetition, and validation of solutions.
Question 2: Why is data quality so crucial in structured problem-solving?
High-quality data is essential. Inaccurate, incomplete, or inconsistent data can significantly impact the accuracy and reliability of conclusions drawn from analysis. A rigorous approach to data validation and cleaning is critical to ensuring results align with reality. Without accurate data, conclusions risk being misleading and potentially harmful.
Question 3: How does reproducibility contribute to the credibility of findings?
Reproducibility ensures that results can be replicated by others using the same methods and data. Thorough documentation of steps taken enhances the credibility of the process. If a study or analysis can be reproduced, confidence in its validity and significance increases. This allows for review, independent validation, and the wider application of knowledge.
Question 4: What role does team collaboration play in a structured approach?
Team collaboration is pivotal in a structured approach. Shared understanding of objectives, division of labor based on expertise, open communication, and constructive conflict resolution are essential for a cohesive team effort. This approach can maximize the collective knowledge and skills within the group, potentially leading to better problem-solving and results.
Question 5: How do clear criteria contribute to consistent results?
Clear criteria establish specific benchmarks and standards, ensuring consistency and repeatability across different stages of analysis. These criteria provide a defined path, promoting greater precision, objectivity, and reproducibility. This clear definition reduces the risk of subjective interpretation and enhances the validity of outcomes. Without these benchmarks, solutions risk being less consistent and reliable.
Question 6: What are the benefits of employing iterative processes in structured problem-solving?
Iterative processes allow for continuous refinement and improvement throughout the problem-solving journey. Each cycle of analysis builds upon preceding cycles, leading to a more nuanced understanding of the issue. This feedback loop allows for adaptation based on new insights and changing conditions. This dynamic approach helps to produce a more effective and robust solution, leading to better results.
Understanding these frequently asked questions provides a clearer perspective on the importance and practical application of structured problem-solving, especially in data-driven contexts. This section highlights the key components of such an approach and underlines its significance.
The following sections will delve deeper into specific methodologies and practical applications within various fields.
Structured Problem-Solving Tips
Effective problem-solving, particularly within data-driven contexts, often hinges on a structured approach. These tips offer guidance for implementing a methodical process, maximizing efficiency, and ensuring reliable results.
Tip 1: Define Clear Objectives. Establishing precise goals and metrics is crucial. Vague objectives can lead to wasted effort and inaccurate conclusions. For instance, rather than aiming for "increased website traffic," define specific targets such as a 15% increase in unique visitors within the next quarter. This clarity ensures alignment and focus throughout the process.
Tip 2: Gather Comprehensive Data. Collecting relevant and representative data is paramount. Thorough data gathering ensures that analysis is rooted in accurate information. Employ multiple data sources and diverse perspectives to gain a comprehensive understanding of the issue. In market research, this might involve surveying various demographic segments and considering competitor data.
Tip 3: Employ Iterative Analysis. Regular feedback loops and cyclical refinement are vital. Initial analysis should be followed by adjustments based on emerging insights and feedback. This iterative process ensures that the solution adapts to new information and refines the understanding of the problem. For example, an initial model predicting customer churn might be revised based on feedback, improving its accuracy over time.
Tip 4: Establish Robust Validation Metrics. Implementing clear benchmarks and metrics for evaluating progress is crucial. These metrics must be quantifiable and directly related to the defined objectives. In a financial modeling scenario, validation metrics might include comparing model predictions to historical data or external benchmarks.
Tip 5: Document Procedures and Findings. Meticulous documentation of methods, data sources, and analysis results is essential for reproducibility and transparency. This allows others to replicate the process and validate findings, enhancing the credibility of the work. Well-documented procedures allow for a smoother workflow and facilitate the collaboration of different teams.
Tip 6: Foster Collaborative Environments. Effective communication and team collaboration are vital. A shared understanding of objectives, division of labor, and open communication channels contribute to a cohesive and efficient problem-solving approach. Conflict resolution mechanisms should be in place to address disagreements constructively.
These tips, when applied consistently, create a structured framework that enhances the reliability and efficiency of problem-solving, particularly in data-driven environments. A methodical approach improves the accuracy of analysis and strengthens the credibility of conclusions.
The following sections will provide practical examples and applications of structured problem-solving methodologies within diverse fields.
Conclusion
This exploration of structured problem-solving, a critical component in data analysis, has illuminated the multifaceted nature of this approach. The article highlighted the iterative process, emphasizing data collection, validation metrics, clear criteria, reproducibility, team collaboration, and data quality as integral elements. The importance of a well-defined methodology was underscored, demonstrating how a structured approach minimizes errors, improves consistency, and enhances the reliability of outcomes. Each step, from defining objectives to validating results, contributes to a robust and repeatable framework, crucial for producing trustworthy and impactful insights from data.
The principles discussed here extend beyond specific applications and hold broad relevance in various fields. A structured approach to problem-solving fosters a more rigorous and trustworthy analysis process. Applying these principles across diverse contextsfrom scientific research to business analyticscan significantly enhance decision-making and contribute to more impactful outcomes. The ongoing development and adaptation of these methods are essential to ensure ongoing relevance and effectiveness in a continuously evolving data-driven world.
You Might Also Like
David The Edge: Guitarist's Secrets & InfluencesIs Actor Charles Bronson Still Alive? Latest Update
Mariska Hargitay Age: How Old Is The Actress?
Mariska Hargitay's SVU Debut Age - How Old Was She?
Unveiling 43 Million Slot Machines: Jackpot Insights & Strategies
Article Recommendations


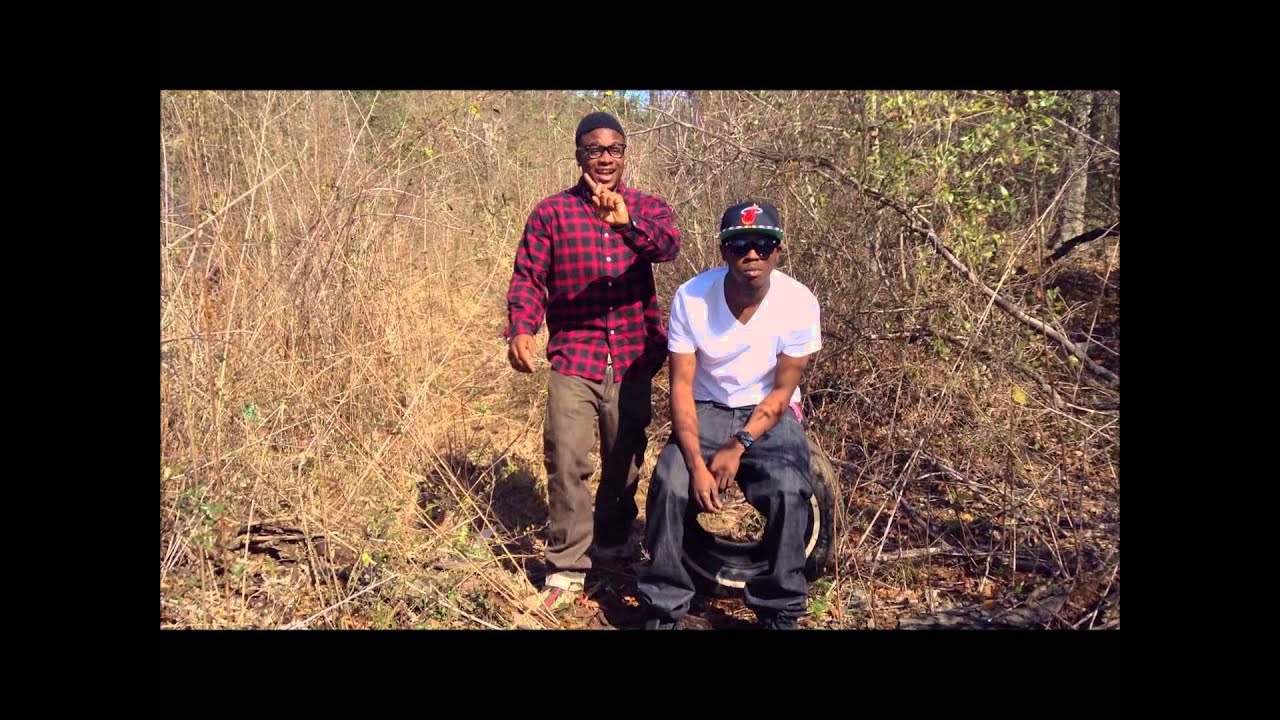